According to a new study about the connection of the AI and Employment from Daron Acemoglu. An Economics Professor at the MIT the advent of artificial intelligence (AI) captivated both the academic and popular imagination. With many hailing it as a transformative technology poised to revolutionize the global economy.
The technology’s rapid developments — particularly in generative AI and large language models (LLM) —sparked debates. These around its potential impact on productivity, employment, and income distribution.
While some forecasts predict explosive growth and productivity gains, others take a more cautious approach. Questioning whether AI’s macroeconomic effects will be as profound as many claim.
This article examines these claims, focusing on the task-based model of AI’s effects and the broader macroeconomic implications.
Task-Based Model of AI
At the heart of the paper’s analysis is a task-based model, which explains how AI impacts the economy by
- automating tasks and
- complementing human labor.
The model rooted in the idea that production involves performing a series of tasks. Which allocated either to labor (human workers) or capital (machines, including AI).
Automation involves AI taking over tasks previously performed by humans. While task complementarities refer to AI improving human productivity in certain tasks without fully replacing workers.
The model draws on Hulten’s theorem. This states that the macroeconomic effects of AI can be estimated by looking at two factors:
- The fraction of tasks impacted by AI (either through automation or complementarities).
- The average cost savings or productivity improvements at the task level.
In this context, productivity gains at the microeconomic level (within individual tasks) translate into broader macroeconomic consequences for
- total factor productivity (TFP) and
- gross domestic product (GDP).
The study uses this framework to evaluate AI’s potential impact on productivity and GDP growth over the next decade – drawing on various empirical studies.
Modest Macroeconomic Gains from AI
Despite the widespread excitement around AI, the paper argues that AI’s macroeconomic effects are likely to be more modest than many expect.
Based on current estimates of the fraction of tasks exposed to AI and the expected productivity improvements. The paper calculates that AI could lead to no more than a 0.71% increase in total factor productivity (TFP) over the next 10 years.
This translates into an approximate annual increase in TFP growth of 0.07%, which is far below some of the more optimistic forecasts.
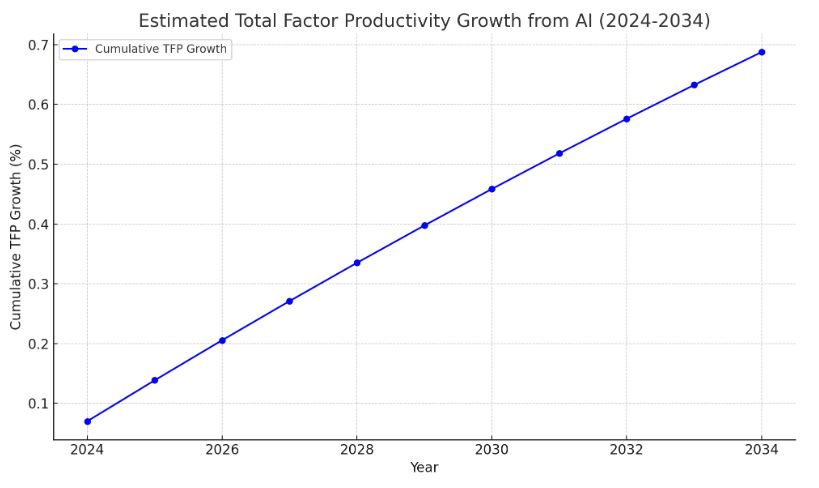
The chart estimating cumulative Total Factor Productivity (TFP) growth from AI over the next 10 years, from 2024 to 2034. The chart shows a modest increase in productivity. Reflecting the prediction of a 0.55% to 0.71% total growth in TFP over the decade due to AI.
This estimate is derived from studies like Eloundou et al. (2023) and Svanberg et al. (2024). This provide data on the proportion of tasks in the U.S. economy that are exposed to AI and the cost savings associated with these tasks.
By aggregating these task-level impacts across the economy, the paper arrives at a modest overall productivity gain. This suggests that while
- AI’s microeconomic effects may be significant within certain industries or tasks,
- AI’s aggregate macroeconomic impact will likely be limited, at least in the medium term.
Overestimation of AI’s Impact
The paper also cautions that even these modest estimates may be overinflated.
Simple tasks AI master
A key reason for this is that many of the early productivity gains from AI have come from “easy-to-learn” tasks — tasks that are relatively simple for AI to master due to their repetitive and objective nature.
These include tasks like
- text generation,
- data classification, and
- basic pattern recognition,
which AI systems like ChatGPT and other generative models have excelled at.
Challenges for AI in “hard-to-learn” tasks
However, much of the future potential for AI lies in “hard-to-learn” tasks, where AI faces significant challenges due to the
- complexity,
- context-dependence, and
- subjective nature of decision-making.
These tasks often involve
- tacit knowledge,
- human intuition, and
- the ability to navigate social and emotional factors,
all of which are difficult for AI to replicate.
The paper argues that productivity gains in these more challenging tasks will be much lower than in easy tasks, further tempering expectations for AI-driven growth.
Incorporating these considerations, the paper revises its estimates downward, predicting that TFP growth from AI will likely be less than 0.55% over the next 10 years.
Wage and Inequality Effects
In addition to its impact on productivity and GDP, the paper explores AI’s potential effects on wages and income inequality. One of the key questions surrounding AI is whether it will exacerbate existing inequalities, as previous automation technologies have done.
Historically, automation tended to disproportionately benefit capital owners and high-skill workers, while displacing low-skill workers and contributing to rising wage inequality.
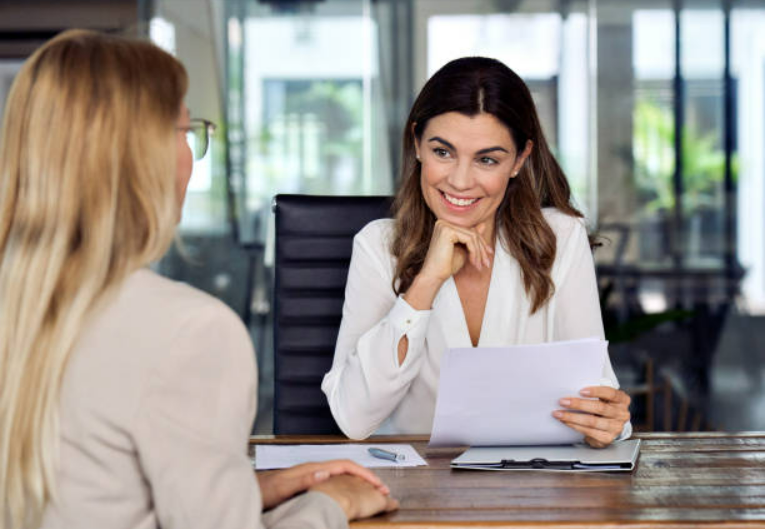
Inequality and productivity won’t be solved
The paper offers a nuanced view, suggesting that AI’s impact on inequality may differ from previous waves of automation. While AI does have the potential to improve the productivity of low-skill workers in certain tasks, this does not necessarily mean it will reduce inequality.
AI’s productivity gains may increase inequality
In fact, the paper argues that in some cases, AI’s productivity gains may increase inequality by disproportionately benefiting certain workers or occupations without creating new tasks for low-skill workers.
Automation could lead to unemploment
For example, if AI automates routine tasks but does not create new job opportunities for displaced workers, it could widen the wage gap between high- and low-skill workers.
Effects of AI in every industry
However, the paper also notes that AI’s impact on inequality may be less severe than that of previous automation technologies. One reason for this is that AI’s effects are more evenly distributed across demographic groups.
Unlike earlier automation waves, which primarily affected manufacturing and routine manual tasks, AI is likely to impact a broader range of occupations, including white-collar and creative roles.
Wage effects and income inequality
As a result, the wage effects of AI may be more evenly spread across the workforce, reducing the risk of dramatic increases in inequality. Despite this more optimistic view, the stufdy concludes that there is little evidence to suggest that AI will significantly reduce labor income inequality.
AI widen the gap in income
Moreover, it predicts that AI will widen the gap between capital and labor income, as the owners of AI technologies are likely to capture a significant share of the productivity gains.
Negative Social Value of New AI Tasks
Another important consideration raised by the research is the potential for AI to create new tasks that have negative social value.
AI could enable harmful activities
While much of the discussion around AI focuses on its potential to enhance productivity and create new economic opportunities, there is also a growing concern that AI could enable harmful activities, as
- the design of algorithms for online manipulation,
- the creation of deepfakes, and
- the proliferation of addictive social media content.
These new tasks, while potentially profitable for certain firms or industries, may have negative consequences for society as a whole.
Negative outcomes of using AI in social media
For example, the widespread use of AI in social media has been linked to negative outcomes as
- increased mental health problems,
- political polarization, and
- the spread of misinformation.
Similarly, AI-powered cyberattacks and security breaches could impose significant costs on the economy, even as they contribute to GDP growth through increased spending on cybersecurity.
The study argues that it is important to account for these negative social externalities when evaluating AI’s macroeconomic effects.
Future research
While AI may boost GDP by creating new economic activities, some of these activities may reduce overall welfare. The paper suggests that future research should focus on developing frameworks to incorporate the negative social value of certain AI-generated tasks into macroeconomic models.
Conclusion
In conclusion, the paper provides a balanced and cautious assessment of AI’s macroeconomic implications.
While AI holds significant promise for improving productivity and transforming the economy, its effects are likely to be more modest than some of the more optimistic forecasts suggest. The task-based model used in the study provides a clear framework for understanding AI’s impact on productivity, GDP, and inequality, highlighting the importance of distinguishing between easy-to-learn and hard-to-learn tasks.
Moreover, the study emphasizes the need to consider the broader social and economic consequences of AI, including its potential to create tasks with negative social value and its complex effects on wage inequality.
While AI may not lead to the explosive economic growth that some have predicted, it will undoubtedly play a significant role in shaping the future of work and the economy.
As AI technologies continue to evolve, it will be essential to monitor their effects closely and develop policies that maximize their benefits while mitigating their potential harms.
Read more about the author on the site of the MIT.